Unlocking the Value of Combination Therapies
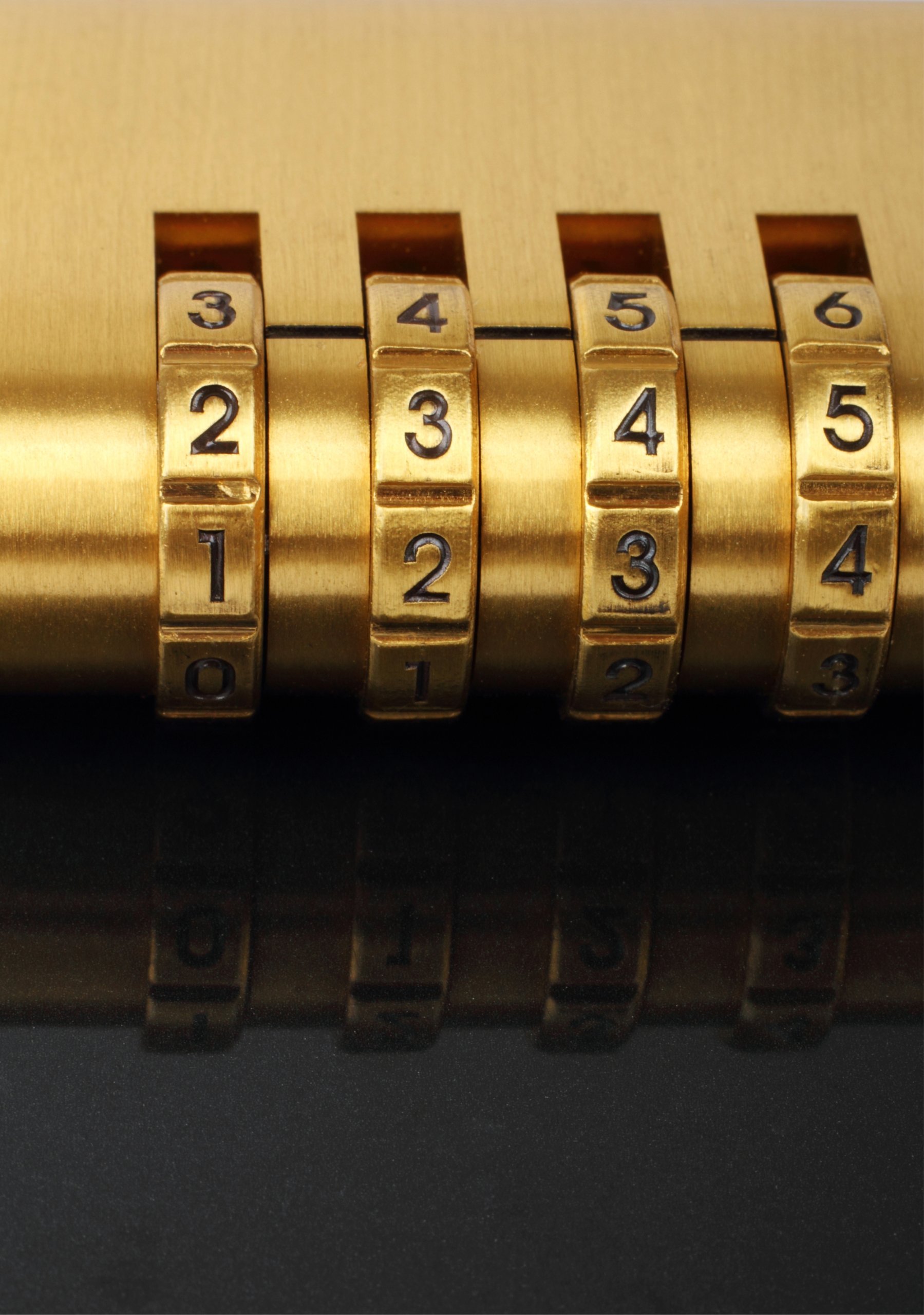
The use of models in economic studies evaluating medicines and other health technologies has become a controversial issue. This is because study results now matter – decision makers are increasingly acting on information about the cost effectiveness of treatments.
This publication is intended…
The use of models in economic studies evaluating medicines and other health technologies has become a controversial issue. This is because study results now matter – decision makers are increasingly acting on information about the cost effectiveness of treatments.
This publication is intended to introduce the key elements of modelling and to explain, with examples, the role that good modelling should play in an economic evaluation. It discusses some of the controversies around the use of models but is not intended to be a guide to this debate. Its purpose is to equip the reader with an understanding of the basic concepts of, and the main uses of, modelling in economic evaluation.
The author, Brian Rittenhouse, argues that we use the term ‘model’ in two quite different ways. In the first sense we take a model to be any artificial simplification of reality designed to enable us to better understand the world. A road map would fall into this category, as would a randomised controlled trial. It is the second meaning of the term model that is more controversial – where the simplification of reality includes the use of techniques to combine data from different sources, and, usually, the use of assumptions to enable extrapolation from the combined data or to fill gaps within the required data set.
After categorising types of model and introducing us to decision analysis, he then addresses the need to have information on effectiveness rather than efficacy. Most randomised controlled trials are designed to demonstrate efficacy, whereas decision makers need to know about effectiveness in clinical practice. This is particularly the case for pharmaceuticals, where pre-launch trials are designed to meet regulatory requirements for safety and efficacy evidence, leading to study designs with high internal validity, but, often, limited external validity. Some of the deficiencies of these trials can, in principle, be dealt with by changes in trial design, others cannot. Modelling can provide a way of turning good efficacy and cost-efficacy studies into good cost-effectiveness analyses.
Of course, concerns about potential bias in data taken from non RCT based studies (see Sheldon (1994)) do have to be addressed, and Rittenhouse discusses sources of bias, hierarchies of evidence, and the extent to which sensitivity analysis and other ways of handling uncertainty can help decision makers understand the potential variation in outcome. He concludes that while sensitivity analysis is valuable it is no substitute for addressing concerns about bias prior to producing the central result of the study.
As Rittenhouse acknowledges, modelling is not without its drawbacks. Readers who wish to follow on from this publication to read more about some of the controversies surrounding the use of modelling, will find a well argued case for the appropriate use of modelling in Luce (1995) and in Gold et al (1996). A thoughtful review of the issues is set out in Buxton et al (forthcoming), and a note of scepticism, restating the case for society to invest on the collection of good evidence from randomised controlled trials, is contained in Sheldon (1996).
Rittenhouse is clear that modelling is a valuable, integral, and permanent feature of economic evaluations and can be of good quality. I hope you find his introduction to the concepts and role of modelling of interest, and that it will stimulate you to read more about modelling techniques and about the debates surrounding its role in economic evaluations and in decision making.
Uses of Models in Economic Evaluations of Medicines and Other Health Technologies
Rittenhouse, B.
(1996) Uses of Models in Economic Evaluations of Medicines and Other Health Technologies. OHE Monograph. Available from https://www.ohe.org/publications/uses-models-economic-evaluations-medicines-and-other-health-technologies/