Socio-Economic Value of Adult Immunisation Programmes
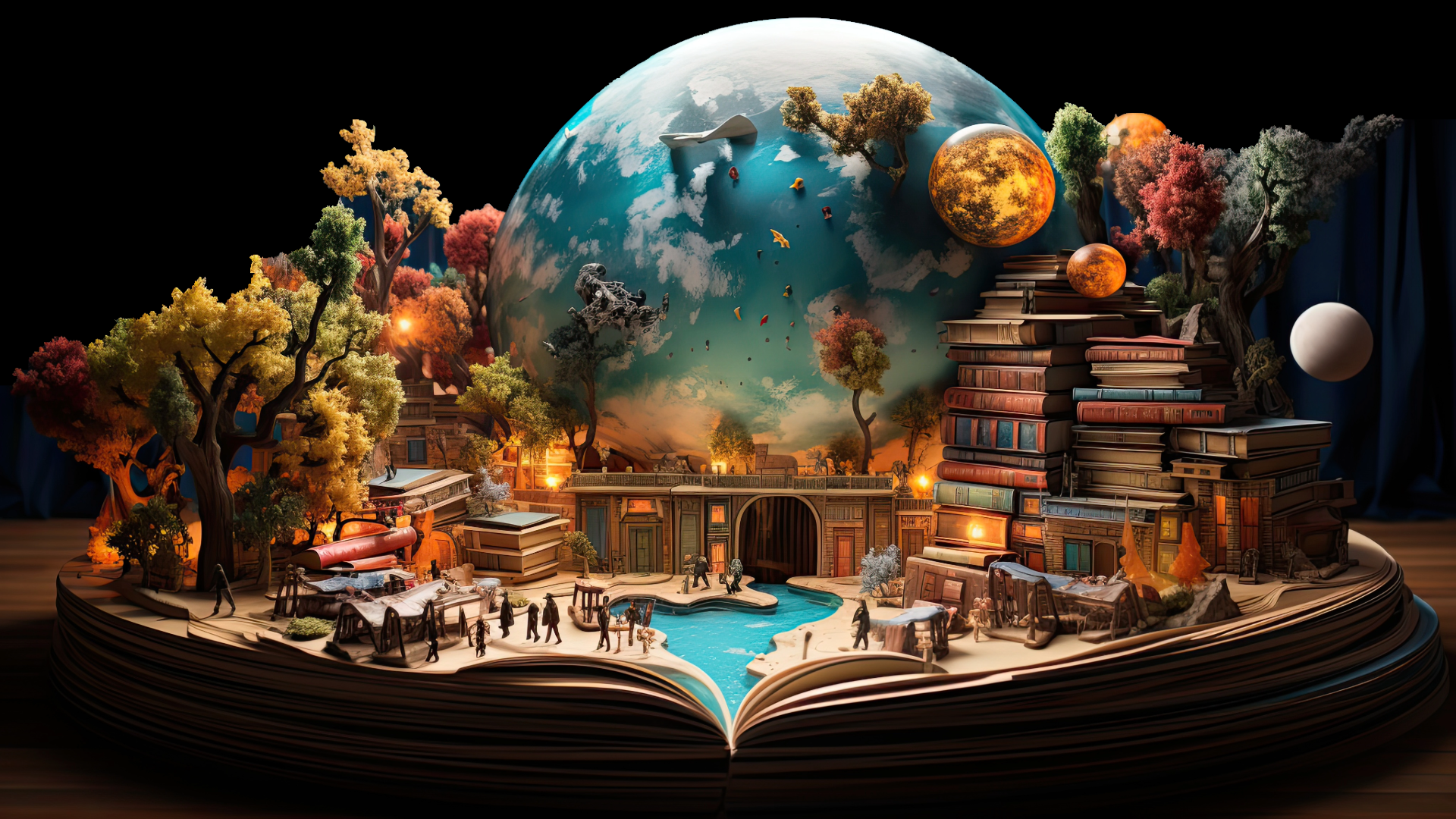
Just published is a new OHE Research paper entitled ‘The Distribution of the EQ-5D-5L Index in Patient Populations’. EQ-5D data are often summarised by an EQ-5D index. The distribution for the original version of the index, the EQ-5D-3L, often shows…
Just published is a new OHE Research paper entitled ‘The Distribution of the EQ-5D-5L Index in Patient Populations’.
EQ-5D data are often summarised by an EQ-5D index. The distribution for the original version of the index, the EQ-5D-3L, often shows two distinct groups in patient populations. This is a result of both the distribution of ill health and how the index is constructed (Parkin et al., 2016). To date, there is little evidence available about the distribution of the EQ-5D-5L index. This project aims to:
Data from Cambridgeshire Community Services NHS’s electronic patient records data warehouse were analysed. EQ-5D-5L profiles before treatment were obtained for 30,284 patients across three patient groups: community rehabilitation services (N=6,919); musculoskeletal therapy services (N=19,999); and nursing services (N=3,366).
The EQ-5D-5L index is calculated using both a ‘mapped’ (crosswalk) value set (MVS) and the English value set (EVS). We examined the distribution of 1,730 of the 3,125 profiles described by the EQ-5D-5L to check for clustering of the EQ-5D-5L index. The k-means cluster method and the Calinski–Harabasz pseudo-F index stopping rule were used to search for the clusters in the index. We examined the impact on the results of using different initial values in the clustering analysis.
Clustering within the EQ-5D-5L index distribution is suggested by both clustering methods, within each of the three patient groups and all patients together. For the all patients’ data, we found two robust clusters for the MVS-based index, compared to three robust clusters for the EVS-based index. The EQ-5D-5L profile data alone do not obviously drive these index clusters.
The results highlight the importance of undertaking careful exploratory data analysis for health related quality of life measures such as the EQ-5D, to ensure that statistical testing takes account of clustering and other features of the data distribution.
The paper was authored by OHE’s Yan Feng, Nancy Devlin, Bernarda Zamora and David Parkin, and Andrew Bateman of the Cambridgeshire Community Services NHS Trust and University of Cambridge.
To access the full paper click here.
This project was funded by a research grant from the EuroQol Research Foundation. The research was also supported by the National Institute for Health Research (NIHR) Collaboration for Leadership in Applied Health Research and Care East of England at Cambridgeshire and Peterborough NHS Foundation Trust.
For more information about OHE’s programme of EuroQol-related research, please contact Nancy Devlin.
An error has occurred, please try again later.
This website uses cookies so that we can provide you with the best user experience possible. Cookie information is stored in your browser and performs functions such as recognising you when you return to our website and helping our team to understand which sections of the website you find most interesting and useful.
Strictly Necessary Cookie should be enabled at all times so that we can save your preferences for cookie settings.
If you disable this cookie, we will not be able to save your preferences. This means that every time you visit this website you will need to enable or disable cookies again.
This website uses Google Analytics to collect anonymous information such as the number of visitors to the site, and the most popular pages.
Keeping this cookie enabled helps us to improve our website.
Please enable Strictly Necessary Cookies first so that we can save your preferences!